With recent scaling of large pre-trained Transformer language models (LLMs), the scope of feasible NLP tasks has broadened. Significant recent work has focused on tasks that require some kind of natural language reasoning. A trajectory in question answering has led us from extraction-oriented datasets like SQuAD to “multi-hop” reasoning datasets like HotpotQA and StrategyQA. Although LLMs have shown remarkable performance on most NLP tasks, it is often unclear why their answers follow from what they know. To address this gap, a new class of explanation techniques has emerged which play an integral part in structuring the reasoning necessary to solve these datasets. For example, the chain-of-thought paradigm leverages explanations as vehicles for LLMs to mimic human reasoning processes. Entailment trees offer a way to ground multi-step reasoning in a collection of verifiable steps. Frameworks like SayCan bridge high-level planning in language and with low-level action trajectories. As a result, we see a confluence of methods blending explainable machine learning/NLP, classical AI (especially theorem proving), and cognitive science (how do humans structure explanations?). This workshop aims to bring together a diverse set of perspectives from these different traditions and attempt to establish common ground for how these various kinds of explanation structures can tackle a broad class of reasoning problems in natural language and beyond.
Schedule
08:00 AM | Virtual Poster Session |
---|---|
08:55 AM | Opening Remarks |
09:00 AM |
Invited Speaker - Sherry Tongshuang Wu How do LLMs Change the Practical Impact of Explanations? [Slides] [Abstract] [Speaker Bio]
Abstract: With the rise of general-purpose LLMs, we also start to think of explanations differently – We now see them less as post-hoc features analyzed by NLP experts and more as a core component of everyday LLM usage for everyone. What exactly do we count as explanations today, and how do we make the best use of them? In this talk, I will review our recent work utilizing and evaluating LLMs in various practical contexts, to reflect on the diverse forms explanations can take and their crucial role in connecting user inputs to final LLM responses. I will also discuss how explanations impact LLM performance, influence user trust and reliance, and emphasize the importance of considering their practical value.
Bio: Sherry Tongshuang Wu is an Assistant Professor in the Human-Computer Interaction Institute at Carnegie Mellon University. Her research lies at the intersection of Human-Computer Interaction and Natural Language Processing, and primarily focuses on how humans (AI experts, lay users, domain experts) can practically interact with (debug, audit, and collaborate with) AI systems. To this end, she has worked on assessing NLP model capabilities, supporting human-in-the-loop NLP model debugging and correction, as well as facilitating human-AI collaboration. She has authored award-winning papers in top-tier NLP, HCI and Visualization conferences and journals such as ACL, CHI, TOCHI, TVCG, etc. Before joining CMU, Sherry received her Ph.D. degree from the University of Washington and her bachelor degree from the Hong Kong University of Science and Technology, and has interned at Microsoft Research, Google Research, and Apple. You can find out more about her at http://cs.cmu.edu/~sherryw.
|
09:45 AM |
Invited Speaker - Karthik Narasimhan Reasoning and Reliability in Language Agents [Slides] [Abstract] [Speaker Bio]
Abstract: As autonomous agents powered by language models enjoy increasing adoption, it is essential to study their robustness and reliability to ensure safe deployment in real-world systems. In this talk, I will present a couple of our recent studies measuring agent capabilities in realistic environments (SWE-bench, τ-bench), and then discuss how 'reasoning' and 'structure' could provide potential solution pathways to open problems in agent performance and reliability.
Bio: Karthik Narasimhan is an associate professor in Computer Science at Princeton and head of research at Sierra. His research spans the areas of natural language processing and reinforcement learning, with the goal of building intelligent agents that learn to operate in the world through both their own experience ("doing things") and leveraging existing human knowledge ("reading about things"). Karthik received his PhD from MIT in 2017, and spent a year as a visiting research scientist at OpenAI in 2017-18 contributing to the first GPT language model. His research has been recognized by the NSF CAREER, an NAE Grainger Foundation grant, a Google Research Scholar Award, an Amazon research award, Bell Labs runner-up prize and outstanding paper awards at EMNLP (2015, 2016) and NeurIPS (2022).
|
10:30 AM | Break 1 |
11:00 AM |
Invited Speaker - Thomas Icard Towards a Pragmatics of Explanation [Abstract] [Speaker Bio]
Abstract: Despite decades of work on the topic, there is still no widely accepted theoretical account of explanation. In the talk we will speculate on why that might be the case, as well as offer a proposal for how a unified account might look, integrating insights from philosophy of science, linguistic pragmatics, and the study of causal cognition.
Bio: Thomas Icard is Professor of Philosophy and Computer Science (by courtesy) at Stanford University. He works at the intersection of cognitive science, philosophy, and computer science, especially on topics related to reasoning, causality and causal inference, decision making, and natural language.
|
11:45 AM |
Oral Presentation: Kartik Chandra, Katherine M. Collins, Will Crichton, Tony Chen, Rachit Nigam, Adrian Weller, Tzu-Mao Li, Joshua B. Tenenbaum, Jonathan Ragan-Kelley WatChat: Explaining perplexing programs by debugging mental models [Abstract]
Abstract: Often, a good explanation for a program's unexpected behavior is a bug in the programmer's code. But sometimes, an even better explanation is a bug in the programmer's mental model of the language or API they are using. Instead of merely debugging our current code (giving the programmer a fish''), what if our tools could directly debug our mental models (teaching the programmer to fish'')?
In this paper, we apply recent ideas from computational cognitive science to offer a principled framework for doing exactly that. Given a ``why?'' question about a program, we automatically infer potential misconceptions about the language/API that might cause the user to be surprised by the program's behavior---and then analyze those misconceptions to provide explanations of the program's behavior. Our key idea is to formally represent misconceptions as counterfactual (erroneous) semantics for the language/API, which can be inferred and debugged using program synthesis techniques. We demonstrate our framework, WatChat, by building systems for explanation in two domains: JavaScript type coercion, and the Git version control system. We evaluate WatChatJS and WatChatGit by comparing their outputs to experimentally-collected human-written explanations in these two domains: we show that WatChat explanations exhibit key features of human-written explanation, unlike those of a state-of-the-art language model. |
12:00 PM |
Oral Presentation: Tianyi Zhang, Li Zhang, Zhaoyi Joey Hou, Ziyu Wang, Yuling Gu, Peter Clark, Chris Callison-Burch, Niket Tandon PROC2PDDL: Open-Domain Planning Representations from Texts [Abstract]
Abstract: Planning in a text-based environment continues to be a significant challenge for AI systems. Recent approaches have utilized language models to predict planning domain definitions (e.g., PDDL) but have only been evaluated in closed-domain simulated environments. To address this, we present Proc2PDDL, the first dataset containing open-domain procedural texts paired with expert-annotated PDDL representations. Using this dataset, we evaluate the task of predicting domain actions (parameters, preconditions, and effects). We experiment with various large language models (LLMs) and prompting mechanisms, including a novel instruction inspired by the zone of proximal development (ZPD), which reconstructs the task as incremental basic skills. Our results demonstrate that Proc2PDDL is highly challenging for end-to-end LLMs, with GPT-3.5's success rate close to 0% and GPT-4o's 38%. With ZPD instructions, GPT-4o's success rate increases to 45%, outperforming regular chain-of-thought prompting's 34%. Our analysis systematically examines both syntactic and semantic errors, providing insights into the strengths and weaknesses of language models in generating domain-specific programs.
|
12:15 PM |
Oral Presentation: Debjit Paul, Robert West, Antoine Bosselut, Boi Faltings Making Reasoning Matter: Measuring and Improving Faithfulness of Chain-of-Thought Reasoning [Abstract]
Abstract: Large language models (LLMs) have been shown to perform better when asked to reason step-by-step before answering a question. However, it is unclear to what degree the model's final answer is faithful to the stated reasoning steps. In this paper, we perform a causal mediation analysis on twelve LLMs to examine how intermediate reasoning steps generated by the LLM influence the final outcome and find that LLMs do not reliably use their intermediate reasoning steps when generating an answer. To address this issue, we introduce FRODO, a framework to tailor small-sized LMs to generate correct reasoning steps and robustly reason over these steps. FRODO consists of an inference module that learns to generate correct reasoning steps using an implicit causal reward function and a reasoning module that learns to faithfully reason over these intermediate inferences using a counterfactual and causal preference objective. Our experiments show that FRODO significantly outperforms four competitive baselines. Furthermore, FRODO improves the robustness and generalization ability of the reasoning LM, yielding higher performance on out-of-distribution test sets. Finally, we find that FRODO's rationales are more faithful to its final answer predictions than standard supervised fine-tuning.
|
12:30 PM | Lunch Break |
14:00 PM |
Invited Speaker - Xiang Lorraine Li Commonsense Knownledge Evaluation for LLMs [Slides] [Abstract] [Speaker Bio]
Abstract: Commonsense knowledge is inherently probabilistic and structured with multiple correct answers. For example, the purpose of “boiling water” could be cooking or making tea. However, people in areas with limited access to clean water might view it as a way to remove germs and ensure it’s safe to drink. Unfortunately, this aspect is often overlooked in the current evaluation of commonsense knowledge. To ensure that models can serve diverse populations, it is important to gather multiple responses from a wide range of people and pay extra attention to rare, yet plausible and important, situations.
This talk will highlight the limitations of current large language models (LLMs) in their commonsense reasoning abilities. I will then discuss two benchmarks for evaluating commonsense in LLMs. One introduces a method for retrieving commonsense question-answer distributions from human annotators, and the other focuses on assessing the long-tail (uncommon) aspects of commonsense knowledge. The new evaluation benchmarks aim to shed light on making LLMs more robust to long-tail knowledge and better catering to diverse populations.
Bio: Xiang Lorraine Li is an assistant professor in the CS department at the University of Pittsburgh. Her research focuses on the intersection of NLP and machine learning, with a particular interest in understanding model behavior through the design of evaluation benchmarks. She explores model performance in complex or long-tail situations, particularly in high-impact domains such as law and education. Her goal is to develop socially responsible, equitable, and robust models that cater to diverse users, populations, cultures, and scenarios. Before joining Pitt, she worked as a young investigator with the Mosaic team at AI2 and completed her Ph.D. in Computer Science at UMass Amherst under the guidance of Andrew McCallum in August 2022.
|
14:45 PM |
Oral Presentation: Jiefu Ou, Arda Uzunoglu, Benjamin Van Durme, Daniel Khashabi The World Is Worth How Many APIs? A Thought Experiment [Abstract]
Abstract: AI systems make decisions in physical environments through primitive actions or affordances that are accessed via API calls. While deploying AI agents in the real world involves numerous high-level actions, existing embodied simulators offer a limited set of domain-salient APIs. This naturally brings up the questions: how many primitive actions (APIs) are needed for a versatile embodied agent, and what should they look like?
We explore this via a thought experiment: assuming that wikiHow tutorials cover a wide variety of human-written tasks, what is the space of APIs needed to cover these instructions? We propose a framework to iteratively induce new APIs by grounding wikiHow instruction to situated agent policies. Inspired by recent successes in large language models (LLMs) for embodied planning, we propose a few-shot prompting to steer GPT-4 to generate Pythonic programs as agent policies and bootstrap a universe of APIs by 1) reusing a seed set of APIs; and then 2) fabricate new API calls when necessary. The focus of this thought experiment is on defining these APIs rather than their executability. We apply the proposed pipeline on instructions from wikiHow tutorials. On a small fraction (0.5%) of tutorials, we induce an action space of 300+ APIs necessary for capturing the rich variety of tasks in the physical world. A detailed automatic and human analysis of the induction output reveals that the proposed pipeline enables effective reuse and creation of APIs. Moreover, a manual review revealed that existing simulators support only a small subset of the induced APIs (9 of the top 50 frequent APIs), motivating the development of action-rich embodied environments. |
15:00 PM |
Oral Presentation: Hyeonbin Hwang, Doyoung Kim, Seungone Kim, Seonghyeon Ye, Minjoon Seo Self-Explore to Avoid the Pit: Improving the Reasoning Capabilities of Language Models with Fine-grained Rewards [Abstract]
Abstract: Training on large amounts of rationales (i.e., CoT Fine-tuning) is effective at improving the reasoning capabilities of large language models (LLMs). However, acquiring human-authored rationales or augmenting rationales from proprietary models is costly and not scalable. In this paper, we study the problem of whether LLMs could self-improve their reasoning capabilities. To this end, we propose Self-Explore, where the LLM is tasked to explore the first wrong step (i.e., the first pit) within the rationale and use such signals as fine-grained rewards for further improvement. On the GSM8K and MATH test set, Self-Explore achieves 11.57% and 2.89% improvement on average across three LLMs compared to supervised fine-tuning (SFT).
|
15:15 PM |
Oral Presentation: Yichen Pan, Dehan Kong, Sida Zhou, Cheng Cui, Yifei Leng, Bing Jiang, Hangyu Liu, Yanyi Shang, Shuyan Zhou, Tongshuang Wu, Zhengyang Wu WebCanvas: Benchmarking Web Agents in Online Environments [Abstract]
Abstract: For web agents to be practically useful, they must adapt to the continuously evolving web environment characterized by frequent updates to user interfaces and content. However, most existing benchmarks only capture the static aspects of the web. To bridge this gap, we introduce Webcanvas, an innovative online evaluation framework for web agents that effectively addresses the dynamic nature of web interactions. Webcanvas contains three main components to facilitate realistic assessments: (1) A novel evaluation metric which reliably capture critical intermediate actions or states necessary for task completions while disregarding noise caused by insignificant events or changed web-elements. (2) A benchmark dataset called Mind2Web-Live, a refined version of original Mind2Web static dataset containing 542 tasks with 2439 intermediate evaluation states; (3) Lightweight and generalizable annotation tools and testing pipelines that enables the community to collect and maintain the high-quality, up-to-date dataset. Building on Webcanvas, we open-source an agent framework with extensible modules for reasoning, providing a foundation for the community to conduct online inference and evaluations. Our best-performing agent achieves a task success rate of 23.1% and a task completion rate of 48.8% on the Mind2Web-Live test set. Additionally, we analyze the performance discrepancies across various websites, domains, and experimental environments. We encourage the community to contribute further insights on online agent evaluation, thereby advancing this field of research. Our platform, tool and dataset are publically available at https://www.imean.ai/web-canvas and https://huggingface.co/datasets/iMeanAI/Mind2Web-Live.
|
15:30 PM | Break 2 |
16:00 PM | In-Person Poster Session |
Speakers
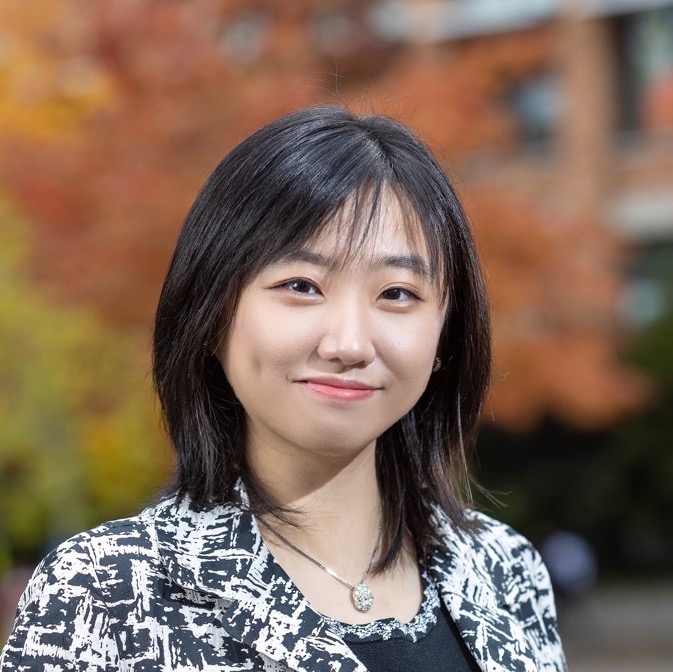
Carnegie Mellon University
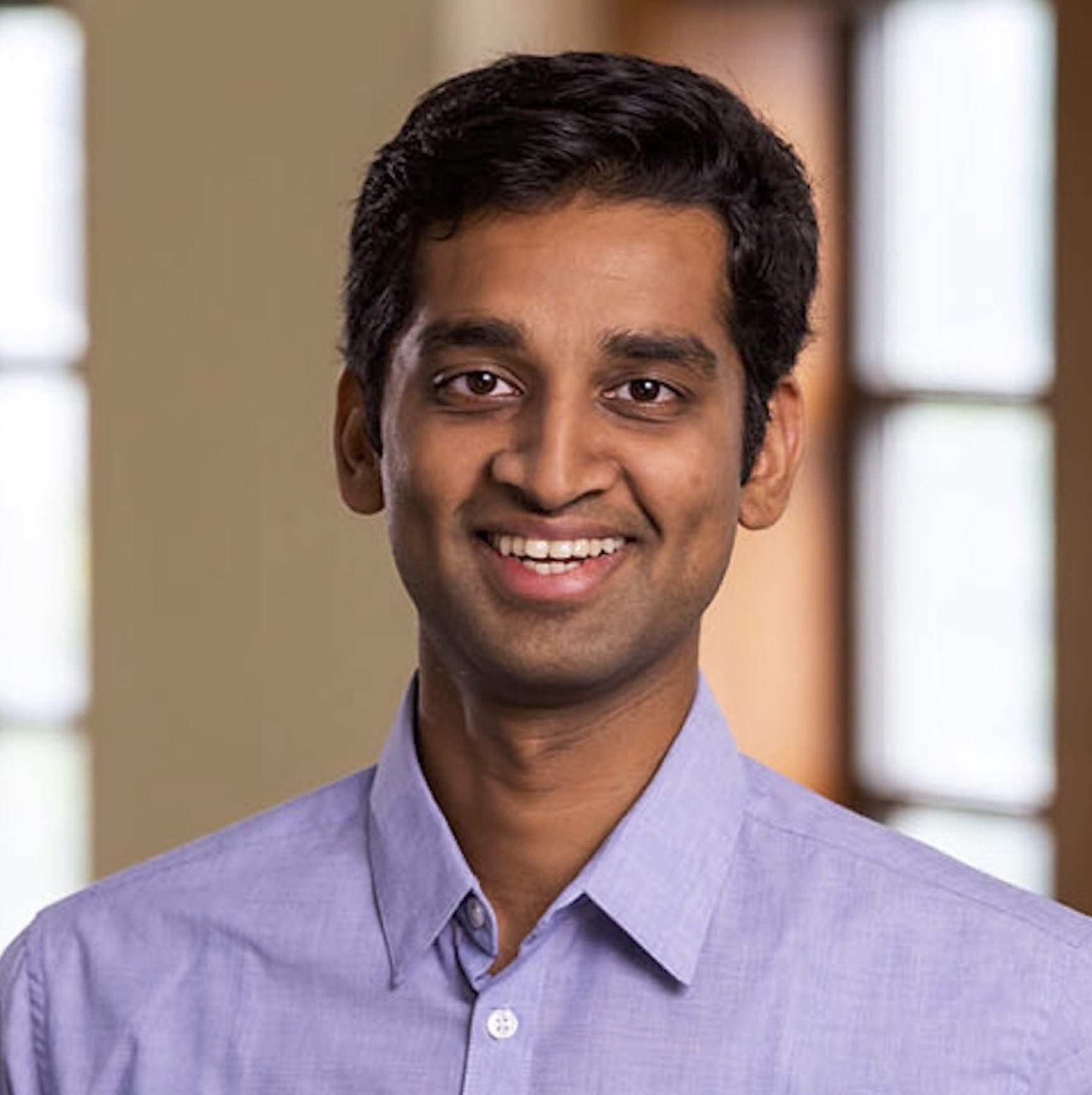
Princeton University
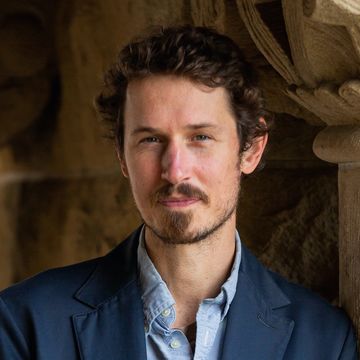
Stanford University
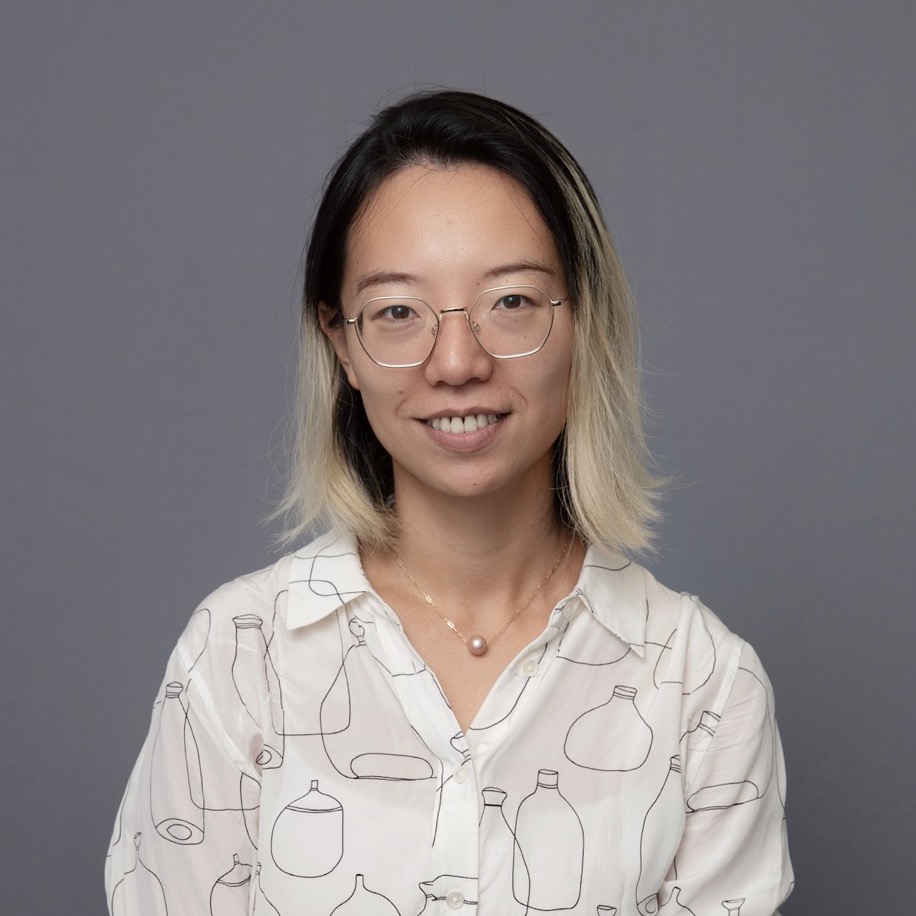
University of Pittsburgh
Accepted Papers
-
Applying RLAIF for Code Generation with API-usage in Lightweight LLMs
[Archival]
-
Are Machines Better at Complex Reasoning? Unveiling Human-Machine Inference Gaps in Entailment Verification
[Cross-Submission]
-
Are self-explanations from Large Language Models faithful?
[Cross-Submission]
-
COPA-SSE: Semi-structured Explanations for Commonsense Reasoning
[Cross-Submission]
-
Concept-aware Data Construction Improves In-context Learning of Language Models
[Cross-Submission]
-
Cooperative Explanation as Rational Communication
[Cross-Submission]
-
Deception in Reinforced Autonomous Agents: The Unconventional Rabbit Hat Trick in Legislation
[Non-Archival]
-
DiffuCOMET: Contextual Commonsense Knowledge Diffusion
[Cross-Submission]
-
Distilling Algorithmic Reasoning from LLMs via Explaining Solution Programs
[Non-Archival]
-
SAGA: A Participant-specific Examination of Story Alternatives and Goal Applicability for a Deeper Understanding of Complex Events
[Cross-Submission]
-
Fill in the Blank: Exploring and Enhancing LLM Capabilities for Backward Reasoning in Math Word Problems
[Non-Archival]
-
From Good to Great: Improving Math Reasoning with Tool-Augmented Interleaf Prompting
[Archival]
-
GraphReason: Enhancing Reasoning Capabilities of Large Language Models through A Graph-Based Verification Approach
[Archival]
-
Inductive or Deductive? Rethinking the Fundamental Reasoning Abilities of LLMs
[Non-Archival]
-
It’s Not Easy Being Wrong: Large Language Models Struggle with Process of Elimination Reasoning
[Cross-Submission]
-
LINC: A Neurosymbolic Approach for Logical Reasoning by Combining Language Models with First-Order Logic Provers
[Cross-Submission]
-
LLMs cannot find reasoning errors, but can correct them given the error location
[Cross-Submission]
-
LOGIC-LM++: Multi-Step Refinement for Symbolic Formulations
[Archival]
-
Language Models as Compilers: Simulating Pseudocode Execution Improves Algorithmic Reasoning in Language Models
[Non-Archival]
-
Large Language Models for Automated Open-domain Scientific Hypotheses Discovery
[Non-Archival]
-
Large Language Models for Automated Open-domain Scientific Hypotheses Discovery
[Cross-Submission]
-
Making Reasoning Matter: Measuring and Improving Faithfulness of Chain-of-Thought Reasoning
[Non-Archival]
-
Multi-hop Question Answering under Temporal Knowledge Editing
[Non-Archival]
-
PARADISE: Evaluating Implicit Planning Skills of Language Models with Procedural Warnings and Tips Dataset
[Cross-Submission]
-
PROC2PDDL: Open-Domain Planning Representations from Texts
[Archival]
-
Prompt Engineering a Prompt Engineer
[Cross-Submission]
-
QA-NatVer: Question Answering for Natural Logic-based Fact Verification
[Cross-Submission]
-
Self-Explore to Avoid the Pit: Improving the Reasoning Capabilities of Language Models with Fine-grained Rewards
[Non-Archival]
-
Self-Training with Direct Preference Optimization Improves Chain-of-Thought Reasoning
[Non-Archival]
-
Small Language Models Need Strong Verifiers to Self-Correct Reasoning
[Cross-Submission]
-
SummEQuAL: Summarization Evaluation via Question Answering using Large Language Models
[Archival]
-
Tailoring with Targeted Precision: Edit-Based Agents for Open-Domain Procedure Customization
[Cross-Submission]
-
TextGenSHAP: Scalable Post-hoc Explanations in Text Generation with Long Documents
[Cross-Submission]
-
The Counterfeit Conundrum: Can Code Language Models Grasp the Nuances of Their Incorrect Generations?
[Cross-Submission]
-
The World Is Worth How Many APIs? A Thought Experiment
[Non-Archival]
-
TimeChara: Evaluating Point-in-Time Character Hallucination of Role-Playing Large Language Models
[Cross-Submission]
-
Towards A Unified View of Answer Calibration for Multi-Step Reasoning
[Archival]
-
WatChat: Explaining perplexing programs by debugging mental models
[Non-Archival]
-
WebCanvas: Benchmarking Web Agents in Online Environments
[Non-Archival]
Call for Papers
We welcome submissions on all topics related to natural language reasoning or structured explanations, which might include:
- Multi-step natural language reasoning;
- Structured explanations;
- Foundations of natural language reasoning;
- Knowledge retrieval for multi-step reasoning;
- Reasoning in interactive environments;
- Applications of natural language reasoning;
- Reasoning as programs;
- Neuro-symbolic reasoning;
With recent scaling of large pre-trained Transformer language models (LLMs), the scope of feasible NLP tasks has broadened, including tasks requiring increasingly complex reasoning. Although LLMs have shown remarkable performance, it is still unclear how to best elicit this reasoning and to what extent the answers that models give follow from what they “know.” This workshop aims to bring together a diverse set of perspectives and attempts to establish common ground for how various kinds of explanation structures can tackle a broad class of reasoning problems in natural language and beyond. As such, the workshop welcomes and covers a wide range of topics, including (non-exclusively):
- Multi-step natural language reasoning: Solving reasoning problems, such as those involving abstract manipulations, has been a long-standing challenge in the field of artificial intelligence. Large language models have recently achieved a new state-of-the-art performance on many reasoning benchmarks, often with approaches only requiring prompting. Current research frontiers are exploring what kinds of explanation formats are most effective, how reasoning is most effectively broken down, how to get language models to plan their reasoning, and what resources can be used to improve reasoning capabilities of language models. Tasks include mathematical reasoning, logical reasoning, commonsense reasoning, and more.
- Structured explanations: Explanations for these complex tasks are typically composed of two or more facts that are used to help guide the reasoning process while also providing a record of the path taken to arrive at an inference. What representations can be best used by inference algorithms to construct large explanations? Frontiers of research include exploring search algorithms over such representations, how to represent annotations at scale and continual learning models.
- Foundations of natural language reasoning: Does the structured reasoning constitute a plausible (interpretable to humans) and faithful (true to the model's processes) explanation? Does perturbing the reasoning lead to correctly modified behavior?
- Knowledge retrieval for multi-step reasoning: It has been shown that LLMs can store factual knowledge implicitly in their parameters, however, their ability to access and manipulate knowledge is still limited. Future avenues of research include effective methods to combine parametric and non-parametric knowledge for complex reasoning, conditioning retrieval given intermediate reasoning context, retrieving better provenance for structured explanations.
- Reasoning in interactive environments: Interactive environments are becoming an increasingly popular method for evaluating reasoning where an agent observes the environment, then takes steps in that environment to accomplish some goal. Here, manner (i.e. how-to) explanations take the form of the list of actions the agent required to accomplish some goal, e.g., "how to boil water in a kitchen", "how to grow an apple tree", "how to book a flight and a hotel in LA".
- Applications of natural language reasoning: New QA settings, language grounding, explainable diagnosis systems, theorem provers using natural language, reasoning for scientific discovery, and more.
- Reasoning as programs: Another body of work within computational cognitive science and AI has formalized reasoning as inference over programs, building on classical views of human reasoning in a symbol-like language of thought and linguistic semantics with logical languages. Language models of code to produce structured reasoning for commonsense problems or other similar approaches are all in scope here.
- Neuro-symbolic reasoning: Pockets of contemporary work have proposed reformulating natural language reasoning as proceeding via modular neurosymbolic systems. Here LLMs operate as declarative programmers, “translating” natural language into a formal specification, such as one accepted by a satisfiability solver, and explicit inference is offloaded to classical symbolic algorithms for planning, constraint satisfaction, or probabilistic simulation.
Submission Guidelines
We welcome three types of papers: archival workshop papers, non-archival papers, and non-archival cross-submissions. Only regular workshop papers will be included in the workshop proceedings. Regular workshop submissions (both archival and non-archival) should be in PDF format and made through the OpenReview website set up for this workshop (link). In line with the ACL main conference policy, camera-ready versions of regular workshop papers will be given one additional page of content. Non-archival cross-submissions should be made through the form (link).
- Archival regular workshop papers: Authors should submit a paper up to 8 pages (both short and long papers are welcome), with unlimited pages for references, following the ACL author guidelines. The reported research should be substantially original. All submissions will be reviewed in a single track, regardless of length. Accepted papers will be presented as posters by default, and best papers may be given the opportunity for a brief talk to introduce their work. Reviewing will be double-blind, and thus no author information should be included in the papers; self-reference that identifies the authors should be avoided or anonymised. Accepted papers will appear in the workshop proceedings. Preference for oral presentation slots in the workshop will be given to archival papers.
- Non-archival regular workshop papers: This is the same as the option above, but these papers will not appear in the proceedings and will typically only receive poster presentation slots. Non-archival submissions in this category will still undergo the review process. This is appropriate for nearly finished work that is intended for submission to another venue at a later date.
- Non-archival cross-submissions: We also solicit cross-submissions, i.e., papers on relevant topics that have already appeared in other venues (e.g., workshop or conference papers at NLP, ML, or cognitive science venues, among others). Accepted papers will be presented at the workshop, with an indication of original venue, but will not be included in the workshop proceedings. Cross-submissions are ideal for related work which would benefit from exposure to the NLReasoning audience. Papers in this category do not need to follow the ACL format, and the submission length is determined by the original venue. The paper selection will be solely determined by the organizing committee in a non-blind fashion. These papers will typically receive poster presentation slots.
In addition, we welcome papers on relevant topics that are under review or to be submitted to other venues (including the ACL 2024 main conference). These papers must follow the regular workshop paper format and will not be included in the workshop proceedings. Papers in this category will be reviewed by workshop reviewers.
Note to authors: For archival and non-archival regular workshop submissions, while you submit your paper through OpenReview (link), please select the "Submission Type" properly based on the guidelines. For cross-submissions, please fill out this form (link) and do NOT submit through OpenReview.
For questions about the submission guidelines, please contact workshop organizers via nl-reasoning@googlegroups.com.
Important Dates
Paper Submission Deadline | May 21, 2024 (All deadlines are 11:59 PM AoE time.) |
Decision Notifications | June 17, 2024 |
Camera Ready Paper Deadline | July 1, 2024 |
Workshop Date | Aug 15, 2024 |
Organizers
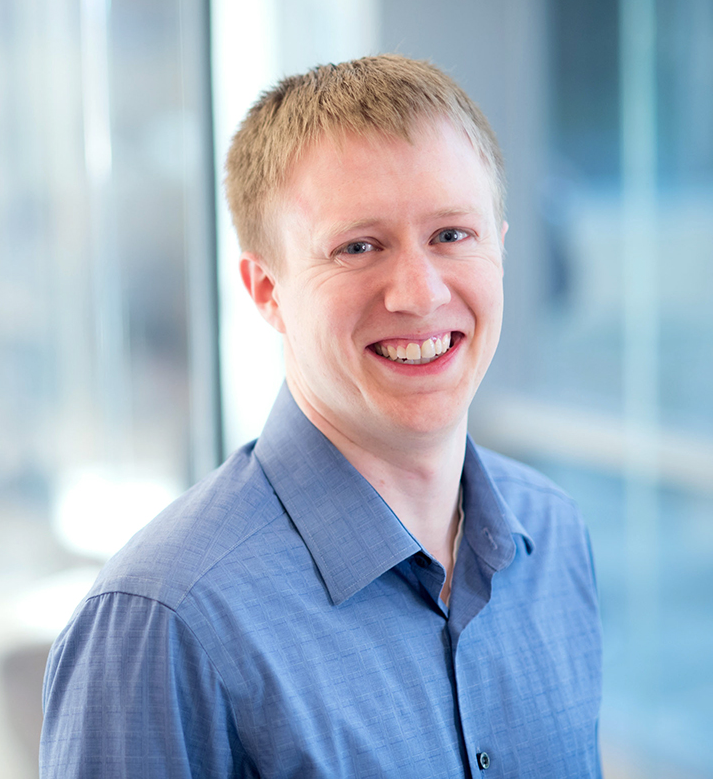
University of Texas, Austin
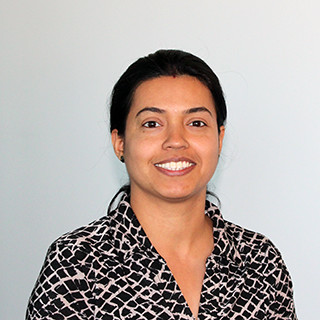
Allen Institute for AI

University of Arizona
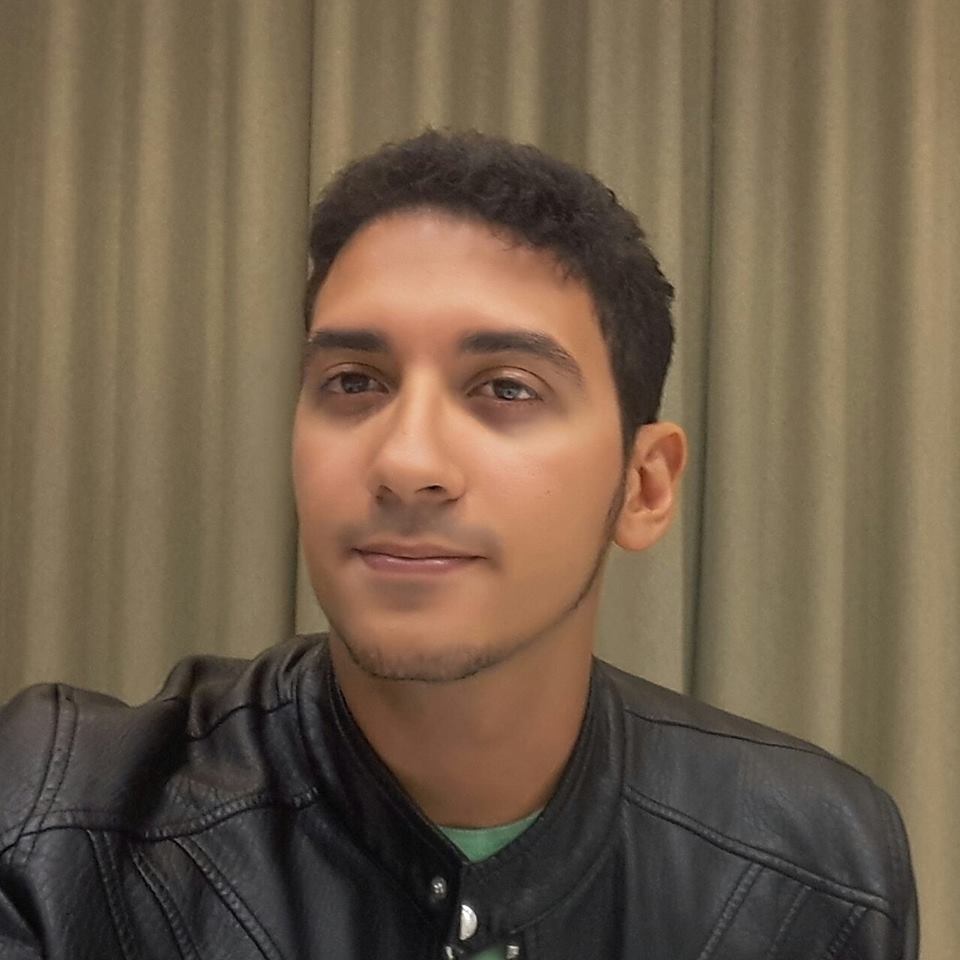
Amazon AWS
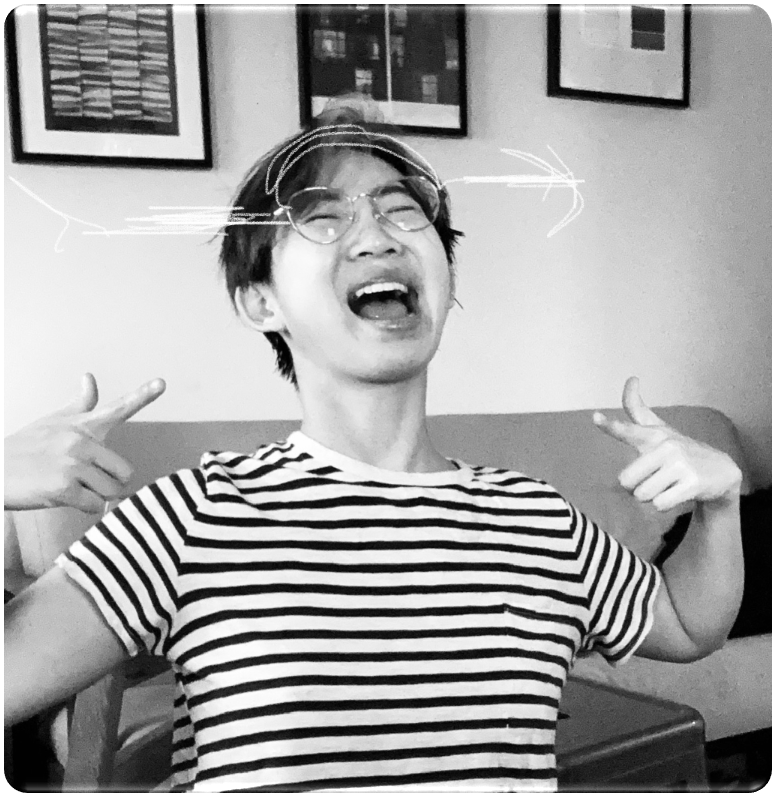
MIT
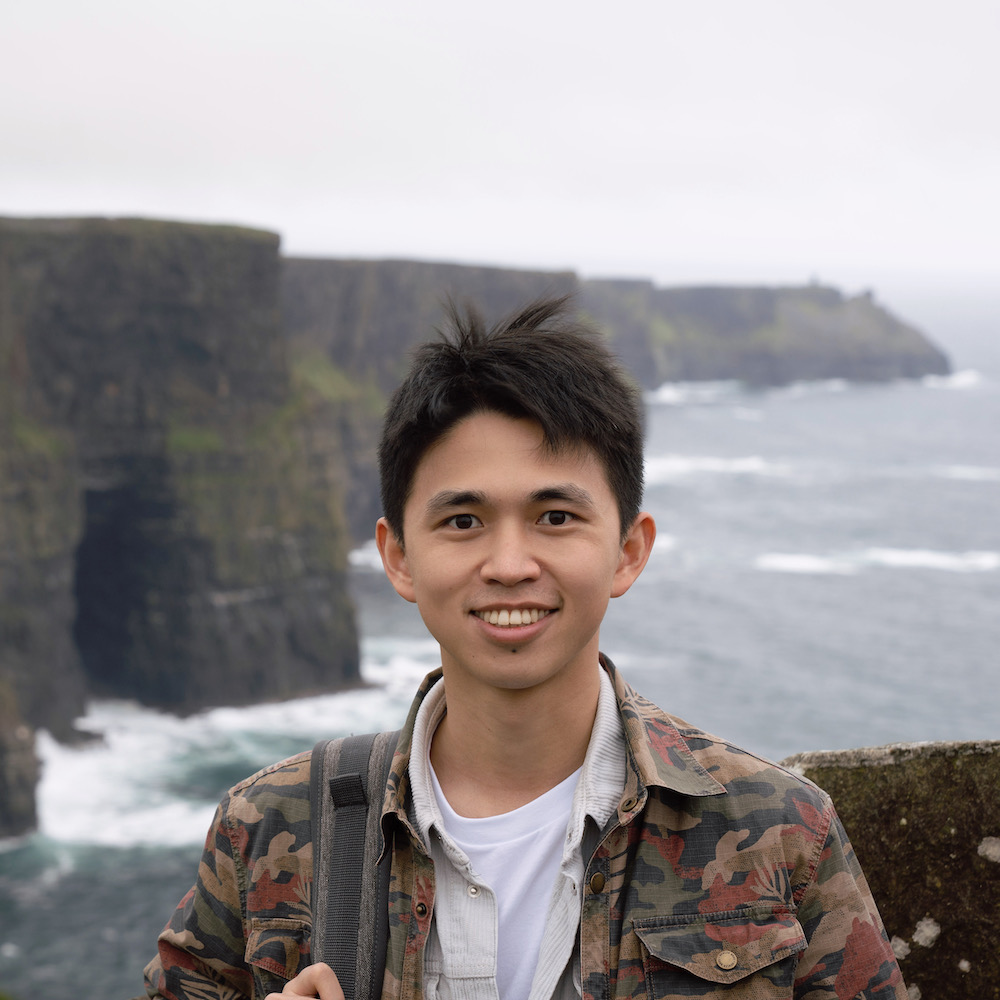
University of Texas, Austin
Cornell University
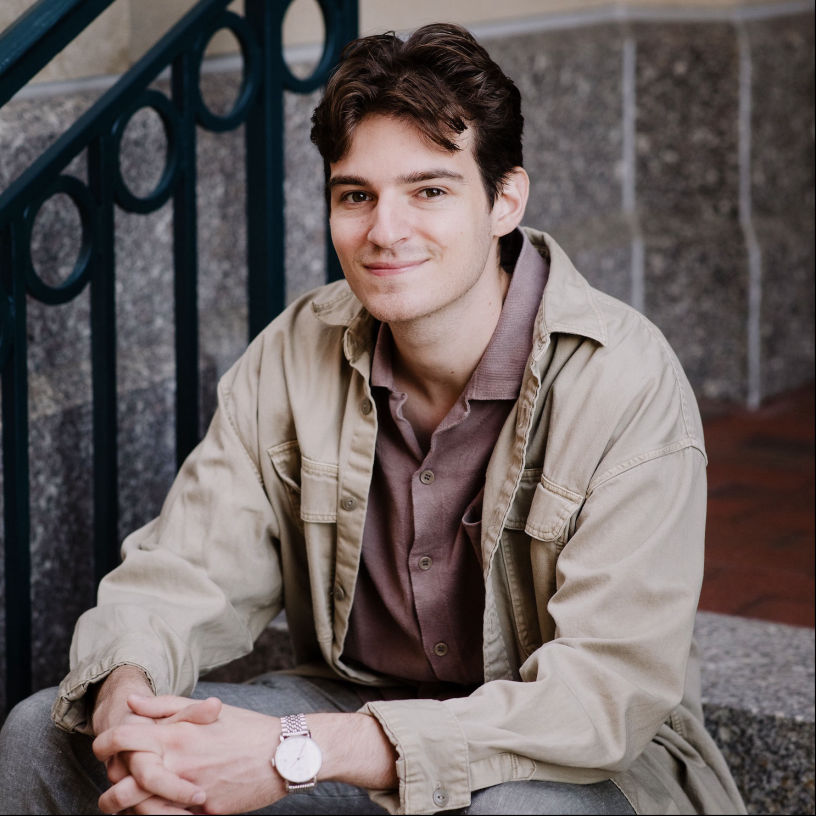